FAIR in the field with FAIMS
Shawn Ross recently was interviewed by Rory Mcneill, for his Fair Data Podcast about FAIMS, digital field data collection, and his professional journey from history to archaeology, open science, and research technology. Rory is the founder of Research Space (an open-source electronic lab notebooks developer and service provider).
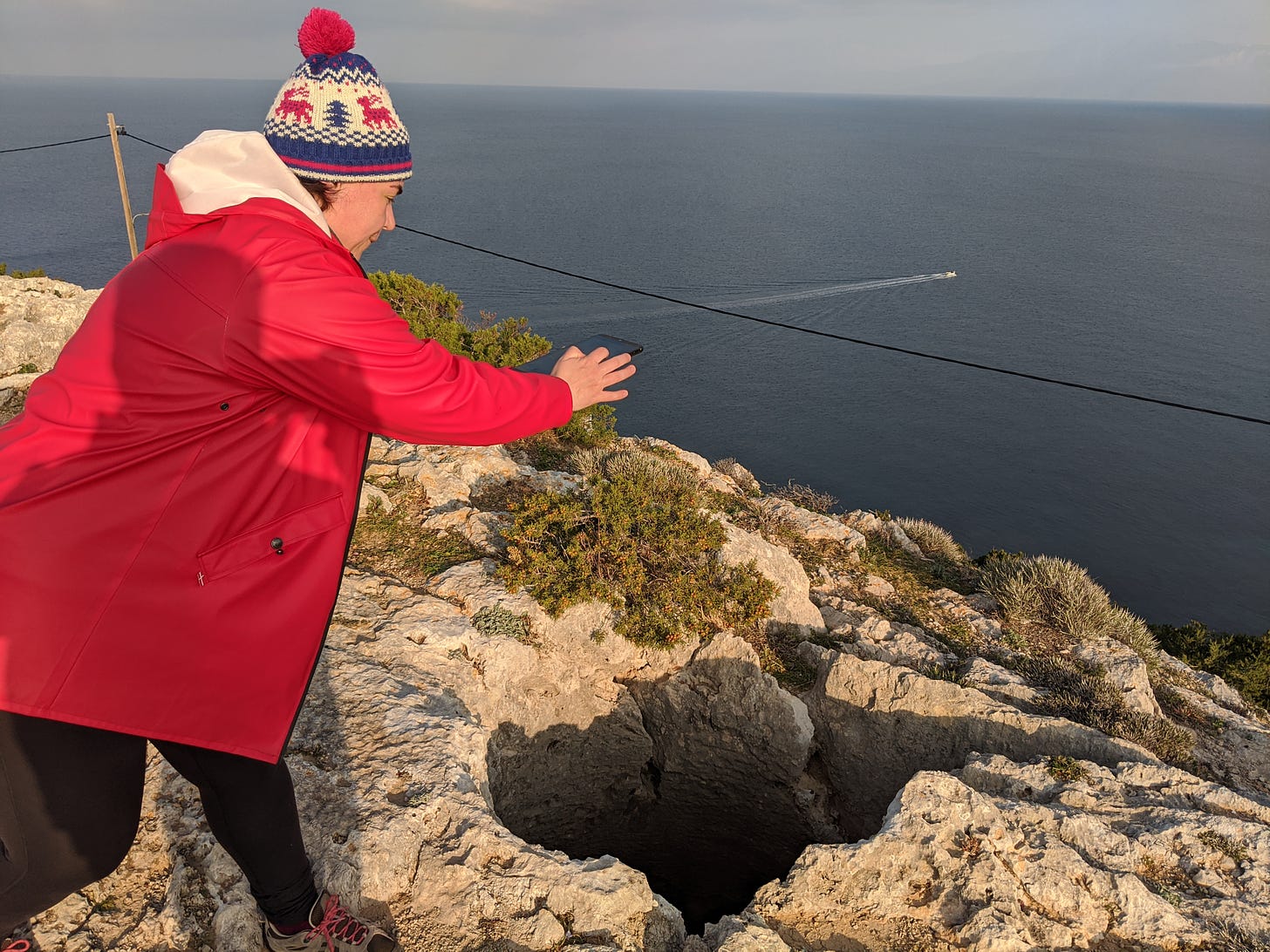
Much of the conversation concerned communicating across boundaries between disciplines and communities of practice, the research information infrastructure environment in Australia, my changing views on research data and data management, and finally the origins and history of the FAIMS Project itself. Rory released the interview on 30 March:
I hope to dedicate future blog posts to expanding on a couple of the topics we discussed, but here I would like to talk about something that Rory and I touched on only briefly in the interview: why it is important to develop field data recording systems that promote Findable, Accessible, Interoperable, and Reusable (FAIR) data in the field.
Last year, several members of the FAIMS team joined a paper in the Journal of Field Archaeology that summarised some reasons for making data FAIRer during fieldwork, as part of an in-depth review of two FAIMS Mobile deployments at the Perachora Penninsula Archaeology Project (PPAP) in Greece. More recently, we’ve started work on a new paper offering a more focused and thorough treatment of the subject (more on that below).
Publishing research data is essential if outsiders are going to evaluate its arguments, claims, and outcomes. Such evaluation is critical, since it has become clear that much, if not most, research findings are false (see for example research assessments from 2005, 2011, 2014, 2015, 2017, and 2021). Although most large-scale analyses of the reliability of research have been undertaken in laboratory or clinical sciences, there is no reason to believe that the situation is any better in social sciences, or other disciplines for that matter. The situation is dire enough that it is now widely known as the ‘reproducibility crisis’ or ‘replication crisis’ — although in the case of disciplines that regularly employ inductive research that seeks to produce rather than prove a hypothesis, it is probably better to speak in terms of research ‘transparency’ instead of reproducibility or replication (noting important work done by Ben Marwick on computational reproducibility in archaeology).
The underlying data is necessary, if not sufficient, to assess the quality of research findings. To use that data to evaluate research, however, you have to be able to find it, understand it, re-analyse it, and otherwise manipulate it. That’s where FAIR comes in. The FAIR principles encourage researchers to:
Describe datasets well enough they can be discovered via manual and automated searches (provided they are placed in appropriate repositories),
Publish data in a way that allows it to be read by both humans and machines,
Licence data so that reuse is allowed, and
Facilitate understanding of data by documenting it with ‘metadata’ (data about the data) and providing a clear story of the origin and history of the data (‘provenance’).
It is much easier to produce FAIR data if FAIRness is ‘built in’ from the beginning, rather than ‘bolted on’ at the end. During fieldwork in particular, it is important for metadata to be produced alongside the data and attached to it. If, for example, the location, environmental context, field conditions, time, date, research method, and record author associated with the collection of a soil sample or the recording of artefacts during archaeological surface survey are not recorded when the data is created, at least some of this information will probably be lost. Without it, the associated data is much less valuable.
Pushing the creation of FAIR data earlier in the research lifecycle to avoid the loss of context is a priority for FAIMS. Both the previous and forthcoming versions of the software incorporate features supporting FAIR elements, like the early application of unique identifiers to records, the automatic or manual (and validated) collection of key metadata, the application of explicit data models (a byproduct of producing a FAIMS customisation), use of controlled vocabularies, and cross-referencing within and between datasets.
Between the podcast I did with Rory, and working on a ‘FAIR in the Field’ paper for a special issue of the Journal of Computing Applications in Archaeology (‘Computer applications and quantitative methods in Australasian archaeology’ - stay tuned for updates!), I’ve been given the opportunity to reflect on what we’ve learned from working with dozens of projects in so many disciplines across the past 10 years(!) of FAIMS development and deployments. I’m looking forward to integrating that experience into FAIMS 3.0 so that researchers can create FAIRer data more easily during fieldwork, and pushing the ‘FAIR in the Field’ envelope further as we deploy the new system.
For more news, subscribe!